101 Ways to Invest in AI-Powered Analytics to Drive Data-Driven Decision-Making in 2025


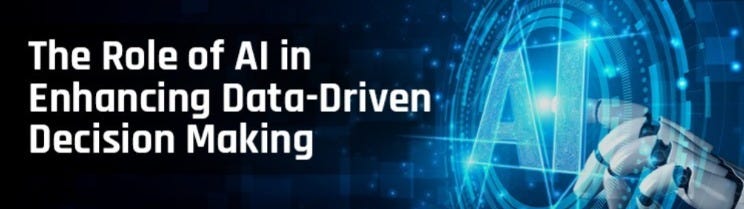
101 Ways to Invest in AI-powered Analytics to Drive data-driven Decision-Making in 2025
Introduction:
The data revolution is in full swing, and organizations are increasingly recognizing the power of data-driven decision-making. In 2025, AI-powered analytics will be paramount, transforming how businesses operate and compete. This guide explores 101 ways to invest in this transformative technology, providing a roadmap for organizations seeking to leverage AI for enhanced insights and strategic advantage. This isn’t just about adopting technology; it’s about building a data-driven culture.
Objectives:
- To provide a comprehensive overview of investment opportunities in AI-powered analytics.
- To highlight the potential benefits and challenges of these investments.
- To offer practical guidance for organizations seeking to implement AI-driven analytics strategies.
- To explore the future trends and emerging areas in AI analytics.
Importance:
Investing in AI analytics is crucial for several reasons:
- Enhanced Decision-Making: AI provides deeper insights from complex datasets, leading to better-informed decisions.
- Increased Efficiency: Automating analytical tasks frees up human resources for more strategic initiatives.
- Improved Customer Experience: AI enables personalized experiences and targeted marketing.
- Competitive Advantage: Organizations that effectively leverage AI analytics gain a significant edge.
- Unlocking New Revenue Streams: AI can identify new market opportunities and drive innovation.
Purpose:
The purpose of this guide is to serve as a practical resource for:
- Business leaders seeking to understand the value of AI analytics.
- IT professionals were responsible for implementing AI solutions.
- Investors looking for opportunities in the AI analytics market.
- Data scientists and analysts seeking to expand their knowledge.
An Overview of Profitable Earning Potential:
The earning potential from AI-powered analytics is substantial and multifaceted:
- Cost Reduction: Optimizing operations, reducing waste, and preventing fraud.
- Revenue Growth: Increasing sales through targeted marketing and personalized recommendations.
- New Product and Service Development: Identifying opportunities for innovation and creating new revenue streams.
- Increased Market Share: Gaining a competitive advantage through superior insights and decision-making.
- Improved Customer Loyalty: Enhancing customer satisfaction and retention.
Infrastructure and Tools:
- Cloud Computing Platforms: Invest in cloud providers offering AI/ML services (AWS, Azure, GCP).
- Data Storage and Management: Solutions for efficient data storage, processing, and retrieval.
- AI-Specific Hardware: GPUs, TPUs, and other specialized processors for AI workloads.
- Data Integration and ETL Tools: Streamlining data flow from various sources for analysis.
- AI Development Platforms: Frameworks and tools for building and deploying AI models (TensorFlow, PyTorch).
- BI and Visualization Tools: Enhance data storytelling and insights communication.
AI-Powered Analytics Applications:
- Predictive Analytics: Forecasting future trends and outcomes using AI/ML.
- Prescriptive Analytics: Recommending actions to optimize business decisions.
- Customer Analytics: Understanding customer behavior and preferences.
- Marketing Analytics: Optimizing marketing campaigns and ROI.
- Sales Analytics: Improving sales forecasting and performance.
- Supply Chain Analytics: Enhancing supply chain efficiency and resilience.
- Risk Management: Identifying and mitigating potential risks.
- Fraud Detection: Detecting and preventing fraudulent activities.
- Cybersecurity Analytics: Enhancing threat detection and response.
- Healthcare Analytics: Improving patient outcomes and healthcare delivery.
- Financial Analytics: Optimizing investment strategies and risk management.
- HR Analytics: Improving talent acquisition and employee retention.
Data-Related Investments:
- Data Acquisition: Acquiring high-quality data from various sources.
- Data Quality Management: Ensuring data accuracy, completeness, and consistency.
- Data Governance: Implementing policies and procedures for data management.
- Data Security and Privacy: Protecting sensitive data from unauthorized access.
Human Capital:
- Data Scientists and Analysts: Hiring skilled professionals to build and interpret AI models.
- AI Engineers: Developing and deploying AI applications.
- Data Engineers: Building and maintaining data infrastructure.
- AI Ethics and Governance Experts: Ensuring responsible AI development and deployment.
- Training and Development: Upskilling employees in AI and data analytics.
Strategic Initiatives:
- AI Strategy Development: Defining clear objectives and use cases for AI adoption.
- AI Center of Excellence: Establishing a dedicated team for AI innovation and implementation.
- Partnerships and Collaborations: Collaborating with AI technology providers and research institutions.
- AI-Driven Innovation: Exploring new business models and opportunities using AI.
Specific Technologies:
- Natural Language Processing (NLP): Analyzing text and speech data.
- Computer Vision: Analyzing images and videos.
- Deep Learning: Building complex AI models for advanced analytics.
- Reinforcement Learning: Training AI agents to make optimal decisions in dynamic environments.
Emerging Trends:
- Edge AI: Deploying AI models on edge devices for real-time analytics.
- Federated Learning: Training AI models on decentralized data sources while preserving privacy.
- Explainable AI (XAI): Making AI decisions more transparent and understandable.
- Generative AI: Creating new data, content, and designs.
Expanding on Existing Categories:
- Cloud Computing Platforms (Expanding on #1):
- Serverless AI/ML services for cost-effective experimentation.
- AI model marketplaces and pre-trained models.
- Hybrid cloud solutions for balancing performance and security.
- Data Storage and Management (Expanding on #2):
- Data lakes and data warehouses optimized for AI workloads.
- Real-time data streaming platforms for immediate insights.
- Metadata management tools for improved data discovery and governance.
- AI-Specific Hardware (Expanding on #3):
- Neuromorphic computing for energy-efficient AI.
- Quantum computing for solving complex optimization problems.
- FPGA-based acceleration for specific AI tasks.
- AI Development Platforms (Expanding on #5):
- Low-code/no-code AI platforms for citizen data scientists.
- AutoML tools for automating model development.
- MLOps platforms for managing the AI lifecycle.
- Specific Technologies (Expanding on #32–35):
- NLP for sentiment analysis and social media monitoring.
- Computer Vision for quality control and defect detection.
- Deep Learning for image recognition and natural language understanding.
- Reinforcement Learning for robotics and autonomous systems.
Industry-Specific Applications:
- AI in Retail: Personalized recommendations and inventory optimization.
- AI in Manufacturing: Predictive maintenance and process optimization.
- AI in Agriculture: Precision farming and crop yield prediction.
- AI in Energy: Grid optimization and renewable energy forecasting.
- AI in Transportation: Autonomous vehicles and traffic management.
- AI in Media and Entertainment: Content creation and personalized experiences.
- AI in Education: Personalized learning and adaptive assessments.
- AI in Government: Public safety and service delivery.
Focusing on Data and its Lifecycle:
- Synthetic Data Generation: Addressing data scarcity and privacy concerns.
- Active Learning: Optimizing data labeling efforts for model training.
- Data Observability: Monitoring data quality and identifying anomalies.
- Feature Engineering Platforms: Automating the creation of relevant features for AI models.
Expanding on Human Capital:
- AI Research and Development: Investing in fundamental AI research.
- AI Ethics Training: Educating employees on responsible AI practices.
- AI Literacy Programs: Promoting understanding of AI among the general public.
New Investment Areas:
- AI for Sustainability: Addressing climate change and environmental challenges.
- AI for Social Good: Using AI to solve social problems.
- AI in the Metaverse: Creating immersive and interactive experiences.
- AI-Powered Drug Discovery: Accelerating the development of new medicines.
- AI for Personalized Healthcare: Tailoring treatments to individual patients.
- AI for Accessibility: Making technology more accessible to people with disabilities.
Investment Strategies:
- Venture Capital: Investing in early-stage AI startups.
- Private Equity: Investing in established AI companies.
- Public Equities: Investing in publicly traded companies with AI initiatives.
- AI-focused ETFs: Diversifying investments across multiple AI companies.
- Strategic Partnerships: Collaborating with AI companies on specific projects.
- Acquisitions: Acquiring AI companies to integrate their technology.
Focusing on Specific AI Techniques:
- Graph Neural Networks: Analyzing relationships and networks.
- Time Series Analysis with AI: Forecasting trends and patterns over time.
- Bayesian Networks: Reasoning under uncertainty.
More Granular Applications:
- AI-powered chatbots for customer service.
- AI for email marketing optimization.
- AI for social media management.
- AI for content creation and copywriting.
- AI for code generation and software development.
Focusing on Business Processes:
- AI for process automation and robotic process automation (RPA).
- AI for decision support systems.
- AI for knowledge management.
Ethical and Responsible AI:
- Bias detection and mitigation tools.
- AI auditing and compliance solutions.
- AI explainability and interpretability tools.
Future-Oriented Investments:
- Artificial General Intelligence (AGI) research.
- Neuro-inspired AI hardware and algorithms.
- AI for space exploration.
- AI for scientific discovery.
- Investing in AI safety research to ensure beneficial AI development.
Pros:
- Improved Accuracy and Efficiency: AI can analyze vast amounts of data quickly and accurately.
- Real-time Insights: AI can provide up-to-the-minute information for timely decision-making.
- Automation of Repetitive Tasks: Freeing up human resources for more strategic work.
- Personalized Experiences: Tailoring products, services, and marketing to individual customers.
- Identification of Hidden Patterns: Uncovering insights that would be difficult or impossible to find manually.
Cons:
- High Initial Investment: Implementing AI solutions can require significant upfront costs.
- Data Dependency: AI models require large amounts of high-quality data to perform effectively.
- Lack of Explainability (in some cases): Some AI models can be “black boxes,” making it difficult to understand how they arrive at their conclusions.
- Ethical Concerns: Issues related to bias, privacy, and job displacement need to be addressed.
- Need for Specialized Skills: Implementing and managing AI solutions requires skilled professionals.
Conclusion:
Investing in AI-powered analytics is no longer a luxury but a necessity for organizations seeking to thrive in the data-driven economy of 2025. By strategically investing in the right technologies, talent, and data infrastructure, businesses can unlock significant value and gain a sustainable competitive advantage.
Summary:
This guide has explored the diverse landscape of AI analytics investments, highlighting the potential benefits, challenges, and opportunities. By focusing on data quality, ethical considerations, and strategic implementation, organizations can maximize their ROI and drive meaningful business outcomes.
Suggestions:
- Start with a clear business problem: Don’t invest in AI for the sake of it. Focus on specific challenges you want to address.
- Prioritize data quality: Garbage in, garbage out. Ensure you have clean, accurate, and relevant data.
- Invest in talent: Hire skilled data scientists, AI engineers, and data engineers.
- Embrace a data-driven culture: Encourage data literacy and empower employees to use data in their decision-making.
- Consider ethical implications: Implement responsible AI practices and address potential biases.
- Start small and scale gradually: Don’t try to do everything at once. Begin with pilot projects and expand as you gain experience.
Professional Pieces of Advice:
- Stay up-to-date with the latest advancements: The field of AI is constantly evolving. Keep learning and adapting.
- Focus on explainable AI (XAI) where possible: Transparency is crucial for trust and accountability.
- Build strong partnerships with technology providers: Choose vendors with proven expertise and a strong track record.
- Measure your results: Track key metrics to assess the effectiveness of your AI investments.
- Don’t underestimate the importance of change management: Implementing AI can require significant changes to processes and workflows.
Thank you for reading 101 Ways to Invest in AI-powered Analytics to Drive data-driven Decision-making in 2025
This comprehensive structure provides a complete guide, incorporating all the elements.
No comments:
Post a Comment